
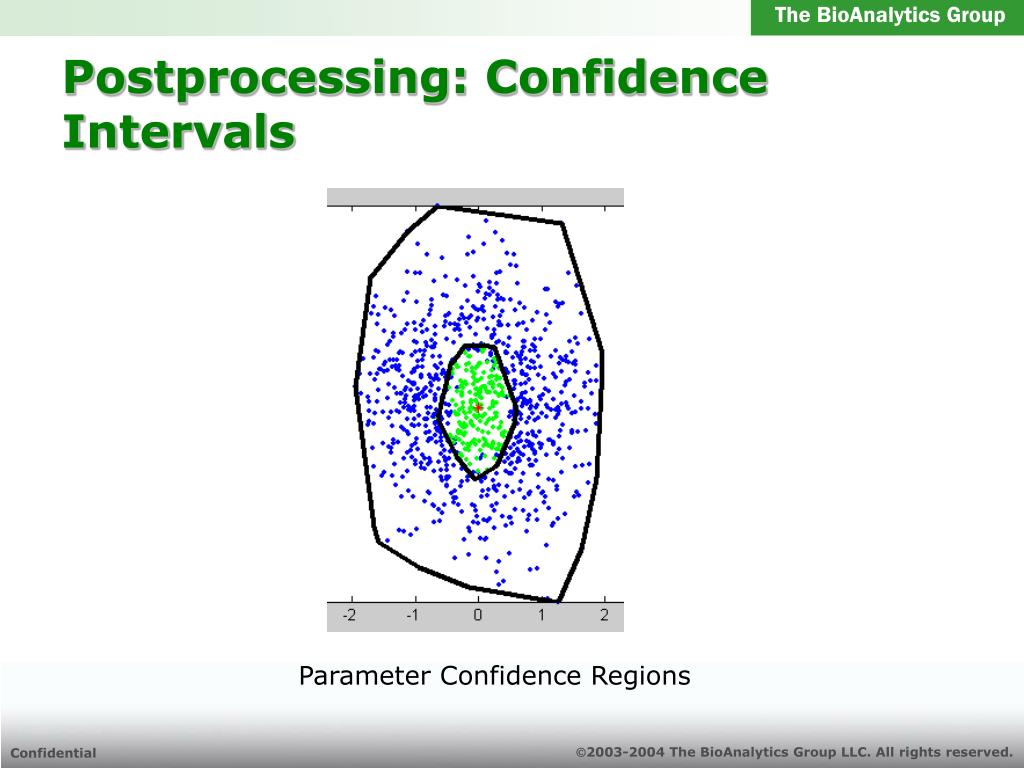
The standard AIPE approach yields the necessary sample size so that the expected width of a confidence interval will be sufficiently narrow. Looking at this formulation, I would guess that this problem becomes analytically intractable quite quickly unless you are okay with union bounding the error probability. Accuracy in parameter estimation (AIPE) is an approach to sample size planning concerned with obtaining narrow confidence intervals. My naive guess as a non-statistician would be that one should set up some kind of optimal Neyman-Pearson like threshold for the $M$ random walks and then let $P($error$)= P(\exists k:\tau_k < \tau_1)$ where $\tau_k$ is the time until walk $k$ reaches this threshold. After searching math.SE and google, I had difficulty finding any literature on this problem, which is kind of surprising given that it seems like a fundamental and interesting problem. I want to identify the parameter $p$ random walk with probability of error less than $\epsilon$ and I want to know how many samples I need to do it (one sample = one observation from each of the $M$ walks). SEQTEST Procedure Perform interim analyses for group sequential clinical trials. The SAS/STAT group sequential design and analysis procedures include the following: SEQDESIGN Procedure Design interim analyses for group sequential clinical trials. Assume that the lifetime of each item has a probability distribution depending on an unknown parameter, where lifetimes with parameter 1 tend to be smaller.
#PARAMETER ESTIMATION BY SEQUENTIAL TESTING TRIAL#
We have $M$ Bernoulli random walks, $X_1$ has parameter $p< 1/2$ and all the rest have parameter $1/2$. If a trial continues to the final stage, the null hypothesis is either rejected or accepted.
